The complete guide to predicting customer behavior by leveraging data
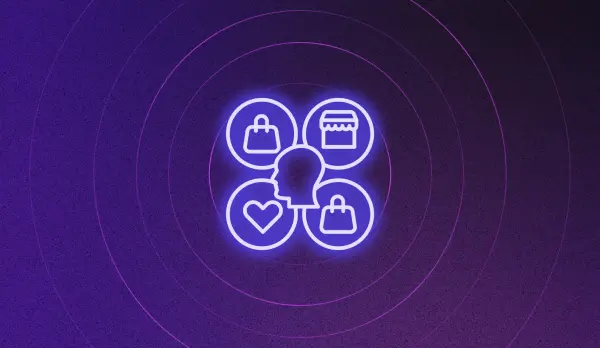
In the rapidly advancing digital age, customer data analysis emerges as a fundamental tool for businesses that want to understand and predict customer behavior. Its relevance is especially pronounced in the dynamic and competitive landscape of ecommerce.
The ecommerce arena is characterized by its fast-paced nature and the ever-shifting consumer preferences, making the need for accurate customer behavior prediction models critical. There are over 44 zettabytes of data in the digital world, so the ability to sift through the massive trove of data from various digital interactions with customers and extract meaningful customer insights is what sets successful businesses apart.
Data analysis enables ecommerce businesses to identify emerging trends, anticipate customer needs, and tailor their offerings accordingly. In 2024, as digital technologies evolve further and consumer behavior becomes more complex, the role of data analysis in crafting business strategies and driving growth will become more prominent than ever.
The role of big data
The concept of big data has transcended from being a buzzword to a critical element in understanding and shaping customer behavior. It refers to the exponentially growing volumes of data generated by digital platforms worldwide.
This data is not only vast in volume but also diverse in variety, encompassing everything from customer transaction records, and social media interactions, to IoT sensor data. Moreover, the velocity at which this data is created and processed is staggering, requiring advanced tools and technologies for its management.
In the context of customer buying behavior, big data serves as a goldmine for consumer insights. It enables businesses to delve deep into consumer preferences, habits, and decision-making processes.
For instance, by analyzing online shopping patterns and social media interactions, companies can understand the factors affecting purchase decisions. Similarly, sentiment analysis of social media data can reveal consumer attitudes toward products, brands, and market trends.
The predictive power of big data lies in its ability to provide a multi-dimensional view of the customer. By integrating data from various sources, businesses can create comprehensive customer profiles and predict future customer behavior with a higher degree of accuracy.
This predictive capability is crucial for personalized marketing efforts, product development, and enhancing customer experiences.
Emerging technologies in data analysis
The landscape of data analytics in ecommerce is being profoundly reshaped by the emergence of artificial intelligence (AI) and machine learning (ML). AI and ML algorithms can sift through vast and complex datasets much more efficiently than traditional statistical methods, uncovering patterns and consumer insights that would otherwise remain hidden.
- AI systems are adept at processing unstructured data, such as customer reviews or social media posts, converting this qualitative information into actionable insights. This capability is especially important in understanding customers' nuanced preferences and sentiments.
- Meanwhile, ML algorithms continuously learn and improve from new data, making them increasingly effective in prediction over time. This dynamic learning process means that the models become more refined and accurate, offering a level of insight into customer behavior that was previously unattainable.
Predictive analytics can be enhanced with deep learning (a subset of ML) to make even more accurate forecasts about customer buying behavior. Additionally, AI-driven natural language processing (NLP) technologies will play a larger role in understanding customer sentiment and feedback, providing businesses with deeper predictive insights into consumer needs and expectations.
The importance of data quality
The effectiveness of data analysis, particularly in the realm of predictive modeling, hinges on the quality of the data used. High-quality data is the foundation upon which reliable predictions are built.
This means the data must be:
- Clean (free from errors)
- Accurate (correct and up-to-date)
- Well-structured (organized in a manner conducive to analysis)
Poor data quality can lead to misguided conclusions and, consequently, ineffective business strategies. Inaccuracies in customer data could result in misinterpreting customer needs, leading to unsuccessful product launches or marketing campaigns. Similarly, outdated data might not reflect current market trends, rendering predictions irrelevant.
As businesses increasingly rely on automated systems for decision-making, the emphasis on data quality will be even greater. Ensuring data integrity involves rigorous processes of data cleaning, validation, and regular updating.
Additionally, data structuring and management practices will play a crucial role in making the data amenable to advanced analytical techniques. Companies that prioritize data quality will be better positioned to leverage the full potential of AI and ML technologies in making accurate and actionable predictions about customer behavior.
Key metrics for customer behavior prediction
Predicting customer behavior accurately requires a deep understanding of various key metrics, each offering unique insights into different aspects of consumer interaction and preferences.
Purchase history
This is a critical indicator of customer preferences and potential future purchases. By analyzing past buying patterns, businesses can anticipate what products a customer might be interested in next.
Browsing patterns
Understanding how customers interact with a website or app (what pages they visit, how much time they spend on each section, and what items they look at but don’t buy) can reveal a lot about their interests and potential buying intent.
Customer feedback
Direct feedback from customers through reviews, surveys, or social media can provide valuable insights into what customers like or dislike about products or services, guiding improvements and innovation.
Engagement levels
Metrics such as time spent on site, repeat visits, email open rates, and social media interactions offer insights into customer loyalty and engagement. High engagement often correlates with higher customer satisfaction and loyalty.
Behavioral indicators
Actions like cart abandonment, frequency of purchases, and response to promotions can help predict future customer actions, such as the likelihood of completing a purchase or responding to a future marketing campaign.
Real-time analysis for dynamic insights
Real-time data analysis allows businesses to gain immediate insights into customer buying behavior, market trends, and operational efficiencies. This immediacy is vital for making quick, informed decisions, as well as for staying competitive.
The instant feedback gathered by real-time analysis allows businesses to immediately gauge the impact of marketing campaigns, product launches, or customer service initiatives, enabling quick adjustments as needed.
When combined with AI and ML, real-time data can be used to predict trends and customer behaviors almost as they occur, allowing businesses to be proactive rather than reactive.
Moreover, real-time analytics can power personalized customer experiences. This can include offering personalized recommendations or discounts to customers as they browse an online store. Also, real-time monitoring of operations can help identify and address issues promptly, reducing downtime and improving overall efficiency.
Key tools for real-time analysis
Here are some analytics tools and technologies that facilitate real-time analysis in customer behavior prediction:
- Data streaming platforms allow businesses to collect, process, and analyze customer behavior and data in real time as it is generated from various sources, such as websites and apps.
- Real-time analytics engines can perform complex analytics operations, such as aggregations, pattern detection, and event forecasting, in real time.
- In-memory databases are essential for scenarios requiring rapid access to data, such as real-time personalization and decision-making.
- Predictive analytics software facilitates the development and deployment of predictive models, analyzes real-time data streams, and provides predictive ecommerce consumer insights almost instantaneously.
- Customer data platforms (CDPs) provide the foundation for real-time analytics by ensuring that the data being analyzed is comprehensive and up-to-date.
- Business intelligence (BI) tools help visualize data in an easily digestible format, enabling businesses to make quick, data-driven decisions.
The ability to analyze data as it is generated will not only provide businesses with a competitive edge but also enhance customer experiences, as businesses will be able to respond swiftly and accurately to customer needs and market changes.
Personalization strategies

The concept of personalization has become a linchpin in modern marketing strategies, driven by an in-depth understanding of customer behaviors. Personalization goes beyond generic offerings to create tailored experiences that resonate with individual customers.
This approach can manifest in various forms:
- Customized product recommendations: Using customer data such as past purchases and browsing history, businesses can recommend products that are more likely to interest individual customers.
- Targeted marketing messages: Personalized marketing communications based on customer preferences, behavior, and previous purchases and interactions can significantly increase engagement rates.
- Dynamic content: Websites and apps that adapt content based on user behavior provide a more engaging and relevant experience, encouraging repeat visits and interactions.
- Personalized customer service: Tailoring customer service based on individual customer profiles and past interactions can enhance customer satisfaction and loyalty.
For example, Starbucks gathers customer data through its rewards programs and mobile applications. With this data, Starbucks tailors product recommendations for its loyal customers, develops more effective marketing campaigns, innovates its menu offerings, and strategically determines locations for new store openings.
Additionally, Starbucks engages in reactivation strategies by sending personalized emails to customers who have not visited in some time. These emails often include special offers and discounts, aiming to rekindle customer interest and encourage store visits.
As technologies evolve, personalization strategies will become even more sophisticated, leveraging AI and ML to create hyper-personalized experiences. This level of personalization not only enhances customer satisfaction but also fosters a deeper, more meaningful connection with the brand.
Predicting customer behavior with data
Predicting customer behavior with data is a multifaceted process that involves several strategic methodologies such as data Integration, segmentation, predictive analytics, and sentiment analysis.
In this process, there are three crucial aspects to consider:
- Customer behavior modeling
- Surveys and social listening
- Brand health assessment
Customer behavior modeling
Customer behavior modeling encompasses a range of techniques and models, each suited to different aspects of customer behavior:
- Heuristic models are rule-based models that apply simple decision rules inferred from empirical data.
- Predictive models use historical data to predict future actions, such as logistic regression models, decision trees, or neural networks.
- Prescriptive models suggest a course of action or strategies based on the predicted outcomes.
- Segmentation models classify customers into different segments based on behavior, preferences, or demographic factors.
Surveys and social listening

Surveys and social listening are essential tools for gauging customer opinions and preferences.
Direct feedback from customers through surveys provides valuable insights into customer satisfaction and expectations. On the other hand, monitoring social media channels for mentions of a brand, product, or industry helps in understanding public sentiment and emerging trends.
Brand health assessment
Brand health assessment is critical for maintaining a positive image in the eyes of customers.
This involves:
- Brand perception analysis: Understanding how customers perceive the brand in terms of quality, reliability, and value.
- Competitive benchmarking: Comparing a brand's performance and health metrics against competitors to gauge relative standing in the market.
- Customer loyalty and retention analysis: Measuring the degree of loyalty and identifying major factors influencing customer retention.
Final thoughts
The intersection of emerging methodologies and advanced technologies has opened up unprecedented opportunities for businesses to understand and anticipate customer needs in ways that were once considered impossible.
The insights gained from predictive analytics are becoming the cornerstone of strategic decision-making in businesses. This shift is not merely about leveraging data for incremental improvements but about fundamentally redefining how businesses interact with their customers.
Companies are now equipped to deliver personalized experiences at scale, predict market trends with greater accuracy, and adapt to consumer needs in real-time. This ability to not only respond to but anticipate customer behavior is a game-changer in a marketplace that increasingly values personalization and agility.
The role of data analysis in predicting customer behavior is set to be more influential than ever in 2024. Businesses that adapt to these changes, embrace new technologies, and uphold high standards of data quality and ethics will be well-positioned to thrive in this new era. The future of customer engagement is data-driven, and the businesses that recognize and act on this trend will lead the way in their respective markets.